How Can AI Be Best Applied to Quantitative Finance?
The AI revolution is already changing wide swathes of society, impacting core institutions like healthcare, education, and criminal justice…
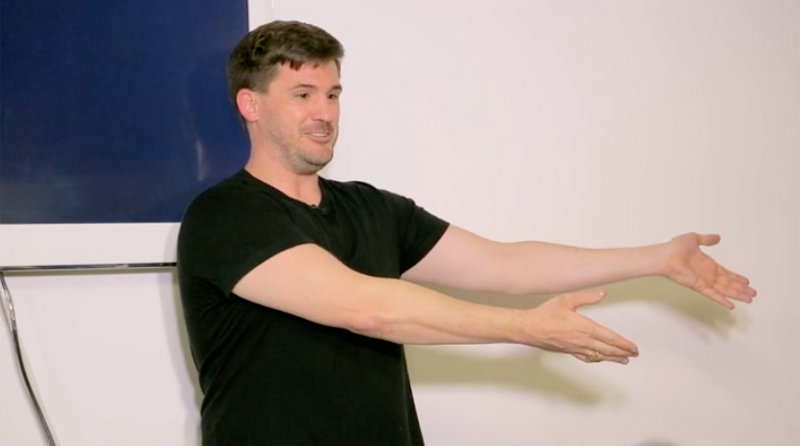
The AI revolution is already changing wide swathes of society, impacting core institutions like healthcare, education, and criminal justice as well as creative industries like music and media. In some sectors, the set of algorithms and technologies that make up AI are just beginning to make their mark. In finance, however, data science techniques (including some machine learning algorithms) have already transformed the world of asset management, changing how sophisticated investors approach portfolio construction and risk management.
The AI Speaker Series at South Park Commons is geared toward understanding how these transformations work by bringing together researchers and practitioners at the forefront of the industry.
Crabel Capital Management’s Justin Nelson, a veteran commodity futures trader, joined the AI Speaker Series at SPC to share his insights into how machine learning has fundamentally altered the financial industry, why quantitative trading models require a new approach to risk, and how investors can make the most of neural networks. His strategies are applicable well beyond the world of machine learning, providing insight into how AI is changing the business world writ large.
Nelson got his start in finance by focusing on risk management, financial modeling, and portfolio theory within consulting firms and hedge funds. In 2012, a Coursera class introduced him to the world of neural networks, and he began experimenting with applying machine learning techniques to building systematic futures strategies. Since then, he has developed robust ways to use machine learning to design trading models and has also become intimately acquainted with the most common challenges and pitfalls with modeling markets.
To Nelson, quantitative trading isn’t simply about maximizing returns — it also requires carefully managing risk. The entry of machine learning into finance means that traders have to be more careful than ever before about how they use and interpret past data and make sure they fully understand the risks they’re taking on. No strategy comes risk-free, and the most successful ones are those that prepare for the unprecedented. Nelson knows what he’s talking about — he has never deployed a financial strategy that lost money.
Here are some of his observations and recommendations:
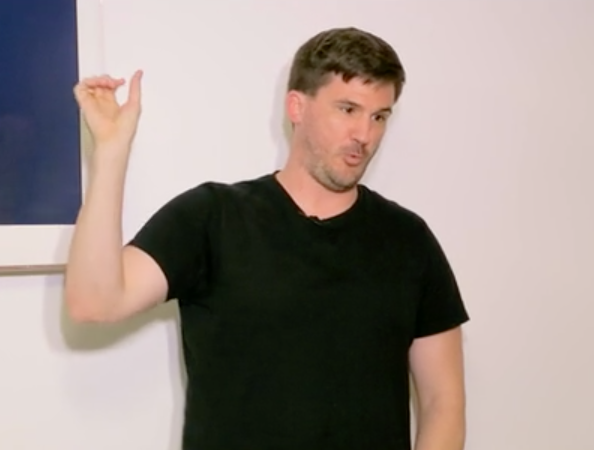
Know your risks
Amateurs think about returns, but professionals think about risks. Separating risk into two categories, beta and alpha, gives traders a better of idea of how exposed their investments will be. Beta risk represents systemic risk in the market (basically what you’re exposed to if you buy into the S&P 500), while alpha represents the idiosyncratic risk left over. Alpha is determined by the independent, “novel” decisions of the trader. Separating the two helps to assess the skill of the “active” decision making involved in a given strategy, and to ensure that systemic risks are well-managed over an entire investor portfolio.
When it comes to maximizing returns, diversification across uncorrelated returns is your friend. “When you come up with a strategy, you have to realize that you’re not the final product,” Nelson said. Rather, you are just a small part of your investor’s portfolio, where the vast majority of their risk tracks equity and bond indices. If most of your portfolio is in stocks and bonds (which provide beta returns), an increase of alpha returns can help your average returns. “Think of risk as a triangle,” Nelson advises. The two bases of the triangle are different risks, while the hypotenuse represents average risk.
Every strategy has a life cycle
Just as life cycles rule the natural world, they also govern the financial world. “You need to constantly be thinking about what’s next, because every strategy will die at some point,” Nelson said. The life cycle of a strategy plays out over several years, because it can take time for investors to come around to it, and still longer for it to approach saturation. Over the course of many years, the life cycle of quantitative strategy typically looks something like this:
- The strategy works
- Capital flows into similar strategies
3. The amount of capital trading the strategy outstrips capacity
4. The strategy dies
5. If sufficient capital flows out of the strategy, the strategy might start working again
In other words, every strategy can live, die, and be resurrected depending on capital flows. “Some of these strategies, they die for several years before they come back,” said Nelson. “It’s a natural cat-and-mouse game.”
Prepare for the unprecedented
Just before the financial crisis, in August 2007, hedge funds began to see unprecedented losses. No one knew what was going on, and they breathed a sigh of relief when things quickly went back to normal within a week. But the abrupt dip was a sign of worse things to come, “the canary in the sub-prime coal mine,” as Nelson put it. There were no historical precedents for the highly-leveraged market conditions of the time, so traders didn’t know how to react. Now, the advent of machine learning in finance means that traders have an even greater imperative to prepare for the unprecedented. Analogy weighting techniques help with basic risk management by emphasizing historical periods with higher proximity scores to today’s data. Having liquid securities with tight stop losses, and using unique modeling inputs can help mitigate risk, but Nelson said that nothing is more effective than simply knowing how your model works. “If you can understand the drivers of performance for the model, you have a chance, a small chance of being able to predict when things are going to break, how they’re going to break,” he said. “It’ll give you a little more confidence to override the model when the conditions are not conducive to what you’re doing.”
Common oversights and mistakes include underestimating trading costs, not understanding the actual risks that are driving performance, Nelson said. Every strategy will work until it doesn’t: “You might have a strategy that works at a very high rate, but when it doesn’t work, it wipes you out,” Nelson said. “The model might not account for a somewhat rare but catastrophic event.” Nelson explains that this is sometimes colorfully described as “picking up pennies in front of a steam-roller.”
Know the signal from the noise
While machine learning can be useful for quantitative trading, as Nelson points out, it can’t be blindly applied because the signal-to-noise ratio in financial time series can be extremely small. “A really good trade wins 55% of the time,” Nelson said. The key to creating a trading strategy lies in first generating a signal, identifying a correlation of prices or a series of events that points toward a given investment strategy.
This means that some popular machine learning methods don’t work — but some do. Nelson shared some of his favored approaches:
- Try bootstrap aggregating, or “Bagging”
Optimize your model by inputting a subset of your data many times, and average the results. This will decrease the model’s variance without increasing its bias. - Use clustering methods
If two securities are historically tightly clustered, bet against significant short-term decoupling. - Build autoencoders
- Look at language
LSTM neural networks can be used to perform sentiment analysis of social media to gauge economic activity, as well as to react to breaking news in real time.
Once you’ve found a signal, you have to allocate the risk associated with your strategy in an intelligent manner and add portfolio-level risk management. Nelson is constantly generating new signals and testing new strategies, the vast majority of which don’t pan out. “You fail a lot. 95% of your ideas don’t work,” he estimated. “You might spend some period of time with an idea that you think is really cool and makes a lot of sense, and then it doesn’t work. You have to think of another idea, you have to constantly be coming up with new approaches.”
Applying machine learning to futures trading comes with a unique set of challenges: one mislabel can skew the entire model, and cleaning up your data can be time consuming and often unsuccessful. What’s more, your model is operating among non-stationary dynamics; market participants are constantly adapting to markets, creating a challenging feedback loop. But neural networks present an unprecedented tool for understanding and mitigating financial risk, taking into account a vast amount of historical data.
Check out the video of Justin’s full presentation at SPC to learn more, and sign up for the SPC email newsletter to be the first to hear about upcoming events in the AI Speaker series.