Accelerating Life Sciences Using AI
"Let's put the machine in the places where we think machines can do a better job."
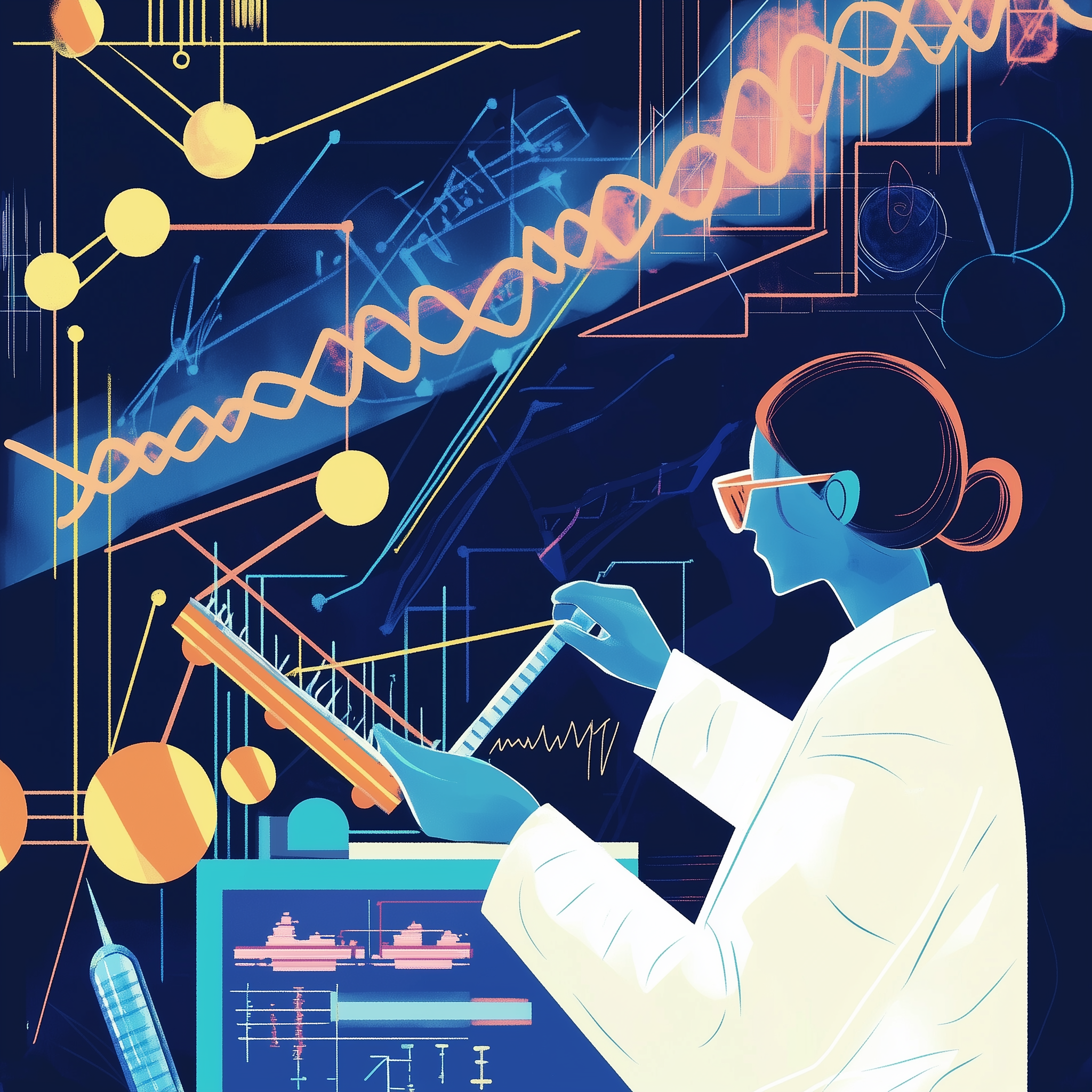
Atray Dixit, current SPC member and founder of Oncko, along with the help of the SPC team, put together a series of lightning talks by executives at top biotech companies who shared insights on how they are using AI to hasten development of live saving therapies through new experiments & resulting discoveries.
We were joined by several industry leaders: Gavin Corcoran, MD, FACP (Chief Development Officer at Formation Bio), Ian Quigley, PhD (CEO at Leash Bio), Peyton Greenside (Founder & Chief Scientific Officer at BigHat Biosciences), Tara Arvedson (Chief Scientific Officer at Hexagon Bio), Ron Alfa (CEO of Noetik), and Harvard University researchers Jonathan Gootenberg & Omar Abudayyeh.
Check out the presentations below!
Formation Bio
Formation Bio is an AI-native drug development company that focuses not on drug discovery, but rather on in-licensing phase two and phase three non-oncology products with existing human data. Unlike many companies using AI for drug discovery, Formation Bio aims to revolutionize the most expensive and time-consuming aspect of drug development: clinical trials. They've already demonstrated success in this area, conducting trials 30-50% faster than industry standards.
The company has formed a strategic collaboration with OpenAI and Sanofi–one of their notable achievements is the development of Muse, an AI recruitment tool that can generate comprehensive clinical trial recruitment materials in multiple languages within minutes, dramatically reducing the time and resources typically required for this process. They're also developing AI tools for asset selection, protocol development, and clinical trial design.
Formation Bio's ultimate vision is to create an "AI scientist" capable of making decisions and reasoning, with humans serving primarily in strategic oversight roles. Their approach involves replacing traditional human-led processes with AI agents where machines can perform better, particularly in areas like toxicity prediction and clinical trial design. The company maintains that this AI-first approach will help achieve their core mission of bringing medicines to patients faster and more efficiently.
Leash Bio
Leash Bio created a project called the Big Encoded Library for Chemical Assessment this past year, a massive dataset designed to predict small molecule-protein binding interactions. Their approach was driven by the observation that successful machine learning solutions typically emerge after the creation of large, high-quality datasets, similar to how ImageNet enabled breakthrough progress in image recognition. Before their project, the largest public dataset for molecular binding contained only about 400,000 interactions – roughly a million times smaller than what was used to train GPT-3.
To address this gap, they generated a massive new dataset of 300 million examples across three proteins using DNA-encoded chemical libraries. The project was remarkably efficient, producing over 4 billion physical measurements from a basement setup with just $40,000 in capital investment. They released this dataset through a Kaggle competition to engage the machine learning community, making all code and data freely available through Polaris.
The team has since scaled up their operations beyond their basement, developing a protein screening engine that processes about 50 new proteins weekly against 6 million molecules. While the initial Kaggle competition revealed the challenging nature of molecular binding prediction–with no contestants able to fully solve the problem–the project represents a significant step toward creating the comprehensive dataset needed to advance this field.
BigHat Biosciences
BigHat is developing next-generation antibody therapeutics that go beyond traditional IgG antibodies, creating what they call "Frankenstein antibodies" – including nanobodies, antibody-drug conjugates (ADCs), and T-cell engagers. While these novel formats offer exciting therapeutic possibilities, they come with significant engineering challenges that traditional monoclonal antibodies don't face, requiring innovative solutions to overcome their inherent liabilities.
The company combines machine learning with synthetic biology in weekly design cycles, using cell-free synthesis to rapidly produce and characterize 2,000 antibodies per week. This approach allows them to evaluate multiple critical properties beyond just binding, including manufacturability, stability, and delivery requirements. Over five years, they've built comprehensive proprietary datasets that inform their specialized models for different aspects of antibody design, from stability to cross-reactivity.
BigHat has progressed to developing clinical candidates, focusing particularly on ADCs and multi-targeted antibodies with sophisticated functionalities. They've achieved notable innovations such as pH-conditional binding (targeting only acidic tumor microenvironments) and molecular logic gates (like NOT gates) that can distinguish between cancer and healthy cells based on target expression patterns. This allows for more precise targeting and potentially higher dosing with reduced toxicity to healthy tissues.
Hexagon Bio
Hexagon Bio is leveraging evolutionary insights to revolutionize drug discovery from natural products. While over 50% of current small molecule drugs are derived from natural products, less than 0.1% of nature's potential has been explored due to the years-long process traditionally required to identify bioactive molecules and their targets. The company focuses on fungi, which are actually closely related to humans, making their bioactive compounds particularly relevant for human therapeutics.
The company's innovative approach centers on identifying resistance genes within biosynthetic gene clusters – the same mechanisms that allow fungi to produce toxic compounds without harming themselves. Using AI algorithms, they analyze their library of 117,000 fungal strains to find biosynthetic gene clusters containing these resistance genes, effectively discovering both the recipe for potential drugs and their targets simultaneously. This is particularly valuable for developing cancer treatments, as these compounds have evolved for "fungal warfare," targeting essential cellular pathways.
Hexagon Bio is currently developing new payloads for antibody-drug conjugates (ADCs), with their first inhibitor targeting protein translation showing promising results against drug-resistant cancer cells. Looking ahead, they're building comprehensive databases linking biosynthetic gene clusters to liquid chromatography mass spectrometry (LCMS) data and 3D structures, aiming to eventually predict a compound's structure and target directly from genetic sequences, dramatically accelerating the drug discovery process.
Noetik
Noetik is tackling the challenge of clinical translation in cancer immunotherapy by developing sophisticated AI models trained on comprehensive patient data. Their approach begins with a full histopathology lab that processes primary human tumor specimens, including over 1,000 non-small cell lung cancer samples, to create "full-stack" datasets that span from tissue-level observations down to genetic information. This includes paired histopathology images, protein measurements, spatial transcriptomics, and whole exome sequencing.
The company complements their human tissue platform with a custom mouse platform that enables high-scaled CRISPR perturbations, allowing them to test multiple genetic knockouts in tumors and draw connections between mouse and human data. Their key innovation is the development of multimodal AI models that can simultaneously process and interpret multiple types of biological data, enabling inferences across different modalities and creating virtual experiments.
One of their notable achievements is the Octo Virtual Cell model, which can predict gene expression based on cellular context and environment. This model enables virtual screening experiments, such as simulating gene knockouts across patient cohorts to identify potential therapeutic targets. Noetik's ultimate goal is to use these models to predict which patients will respond to specific drugs, recognizing that cancer subtypes are defined by distinct biological characteristics rather than just their tissue of origin.
Harvard University
Harvard researchers Jonathan and Omar focus on "programmable biology" across three scales: proteins/molecules, cells, and organisms, with the goal of understanding biological systems programmatically. At the protein level, they've developed a technology called Evolve Pro that uses active learning to understand and optimize protein function, going beyond traditional protein language models to focus on actual functional outcomes rather than just evolutionary fitness.
For cell-level research, they're developing virtual cell models trained on single-cell multiomics data. Their approach makes single-cell RNA sequencing cost-effective (about $10,000 per cell type), enabling them to work toward collecting hundreds of millions of perturbed cells for training. They've created specialized libraries, including one containing 3,500 transcription factors, to probe gene regulatory networks and enhance their foundation models.
The ultimate goal is to accelerate drug development by enabling virtual screening across vast datasets of disease cells (covering 160 different diseases) and aging atlases. This allows them to predict tissue-specific and universal drivers of aging, and identify potential therapeutic targets. They compare their vision to SpaceX's impact on space travel costs, hoping that AI and improved models can similarly transform the economics and efficiency of drug development.
About our emcee: Atray Dixit is an SPC member and the founder & CEO of Oncko. Oncko is a recently formed biotech company focused on tackling the problem of discovering combination therapies. While combination therapies dominate current cancer trials (~70%) and have led to 90% cure rate for certain cancers, most of these efforts have come from rational trial and error. The general problem of finding the right combination has historically been intractable (there are 1.3T ways to select three-way drug target combinations). Oncko has developed >10,000x improved data generation technology to search the under-explored space of combination therapies for cancer. They couple this tech with AI/ML for translating preclinical results into human probability of phase 1 and 2 success predictions. They are now generating some of the largest drug perturbation datasets ever created and validating novel combinations.
Interested in figuring out what to work on next in the most talent-dense technical community around? Apply to SPC below.